By Tom Børsen, Associate Professor, Aalborg University How can we prepare engineering students not only…
Sasha Nikolic, Scott Daniel, and Rezwanul Haque Australasian Association for Engineering Education (AAEE) on Generative AI with Natalie Wint and Neil Cooke
Since the start of 2023, Chat GPT, and the use of generative AI (Gen-AI) more generally, has been the topic of much discussion, advice and debate within engineering education (and indeed education more generally) worldwide. Despite a proliferation of guidance, awareness raising and information, there has been little empirical evidence pertaining to the impact of Gen-AI on integrity of assessment and risk of plagiarism, something which has led to confusion and duplication of work.
In this episode we spoke to Sasha Nikolic (University of Wollongong), Scott Daniel (University of Technology, Sydney), and Rezwanul Haque (University of the Sunshine Coast) from the Australasian Artificial Intelligence in Engineering Education Centre (AAIEEC) Special Interest Group of the Australasian Association for Engineering Education (AAEE), who, along with other Australian engineering educators, came together to answer questions about how ChatGPT and other Gen-AI tools may affect engineering education assessment methods, and how it might be used to facilitate learning.
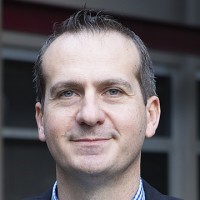
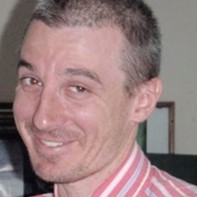
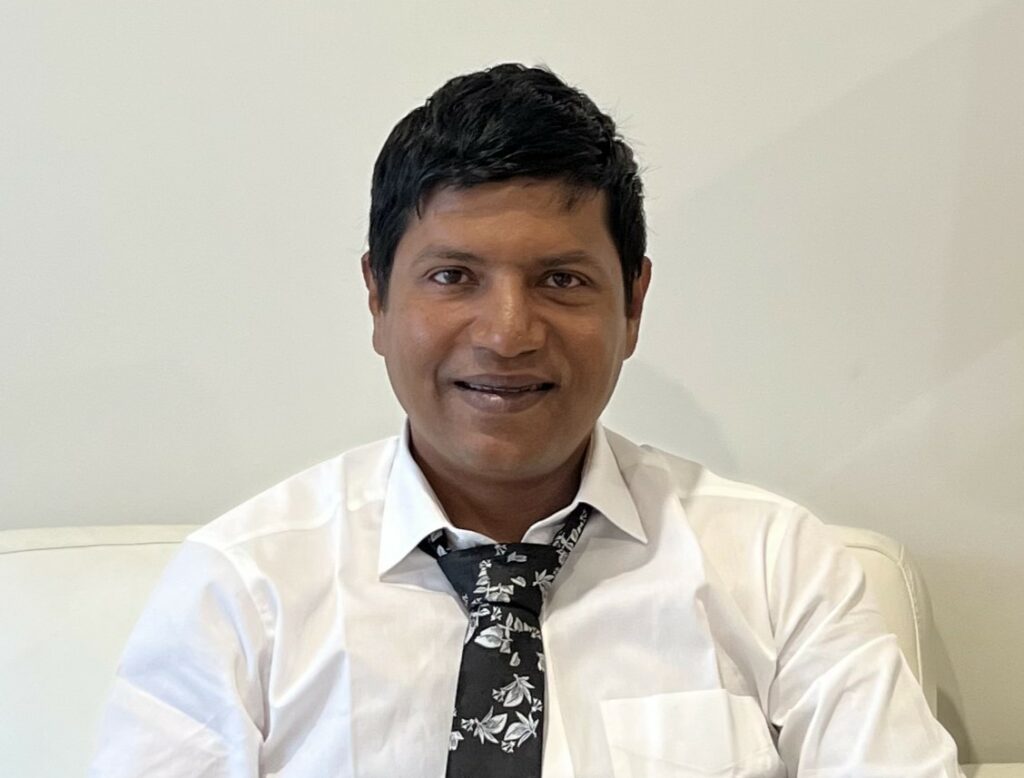
The remainder of this article will summarize discussion points.
AAEE and AAIEEC
The guests outline similarities between AAEE and SEFI in that they both have an annual conference, special interest groups (SIGs) and research training events such as the Annual Winter School. They explain that the AAIEEC has been active for a couple of years, trying to wrestle with the complexity that technological innovation has brought. Although the group is primarily focused on assessment integrity, other aims include the development of AI focused modules and courses; the inclusion of AI within accreditation criteria; opportunities that Gen-AI presents in terms of how we learn and where we can embed it into courses; the ethical implications of AI; fostering diversity and inclusion within AI; and encouraging research collaboration, knowledge exchange and professional development. They explain that over 14 universities and over 40 academic staff are involved in the SIG, something which is important given the resource constraints within higher education. The diversity of institutional contexts and teaching areas also allows for the coverage of many different skills and aspects of the curriculum, as well as assessment types.
Key Terms
The team explain that Gen-AI involves the ability to generate new content such as text, images, music etc. from learnt patterns, and that unlike traditional AI, which is often designed for specific tasks, Gen-AI creates novel outputs that resemble the data its trained on which allows it to predict and generate words, sentences, paragraphs etc. They go on to explain that Chat GPT 3.5 was the first model to cause worry, but that it has since been updated (Chat GPT 4.0). They list some of the other models which include Microsoft Copilot and Gemini (Google), saying that each model has its own strengths, weaknesses and performance levels.
The initial study
Our guests explain that their initial work started out of the panic that resulted from the advent of Chat GPT 3.5, when many questions were asked to engineering education researchers regarding assessment integrity. They set up a project based on integrity of assessment after realizing there was little information available within the literature. The initial study involved 7 universities, 10 researchers and 10 engineering disciplines, which allowed for consideration of different assessment types from different angles and perspectives and for the team to understand the current state of play with respect to the risks posed to different assessment types, as well as the opportunities for integration of Gen-AI into education.
The study assessed the performance of Chat GPT 3.5 against assessment tasks including online quiz, numerical, oral, visual, programming and writing (experimentation, project, reflection and critical thinking, and research).
They explain that the design of the study was based on finding out the minimum amount of effort required to get a pass. The first step was to copy and paste assessment question into Chat GPT 3.5. In a few instances they found it gave the perfect solution. In some cases, the solution provided was close to the actual solution and only minor modification was needed, and in some cases major modification was required.
For example, with online quizzes, correct answers were typically generated when the prompt was the original assessment question. In contrast, in the case of assessments which required higher order thinking skills and evaluative judgement, more modification was needed.
There are multiple implications of this. For example, short term, the team suggest ‘little tricks’ for example placing figures and tables in online quizzes. In the long term they suggest an approach whereby it is assumed that Gen-AI will be used for (and should therefore be integrated into design of any) unsupervised assessment
Improvements and advancements in Gen-AI
The first paper made predictions pertaining to advancements and improvements in Gen-AI models and the team followed up with a second piece of work twelve months later, whereby the original study was repeated using new and updated tools ChatGPT- 4, Copilot, Gemini, SciSpace and Wolfram. The updated study investigated the performance and capability differences, identifying the best tool for each assessment type. The team saw improvements for online quizzes and numerical type work, with multiple benefits associated with the advent of image recognition.
Opportunities
The team talk about several opportunities presented by Gen-AI, one pertaining to open ended problems and ability of Gen-AI to allow students to apply different principles and critical thinking techniques, whereby Gen-AI acts as another team member (co-intelligence). Another opportunity is using Gen-AI as a tutor.
Our guests talk about the need to teach students how to use Gen-AI effectively and the creation of a framework for implementing variants of project work which include use of Gen-AI. For example, you may allow its use for improving the quality of writing but have higher standards in terms of marking criteria. Similarly, you may assess students’ ability to critique or improve outputs of Gen-AI or to make use of code it produces, as opposed to asking them to produce outputs themselves.
They also describe how they have used Gen-AI for the development of question banks.
Takeaway pieces of advice
- If you haven’t yet played around with ChatGPT, book out half an hour and take the time to have a conversation, and copy and paste some of the text from your assessments and see what you get
- Look at your course learning outcomes, spend more time on what you are judging, what the student needs to learn your course so you can redesign your assessment
- Have look at table ten (which provides the ten assessment types, the risk, short and long term options for improving assessment security, opportunities and use cases) from the second paper which provides a starting point into where you need to position yourself in terms of assessment, security and integration. Then move across to the AAEE website where you’ll be able to have a look at a whole range of extra resources.
Resources
For more information about the Australasian Artificial Intelligence in Engineering Education Centre (AAIEEC) Special Interest Group visit: https://aaee.net.au/sigs/
Papers
Nikolic, S., Daniel, S., Haque, R., Belkina, M., Hassan, G. M., Grundy, S., … Sandison, C. (2023). ChatGPT versus engineering education assessment: a multidisciplinary and multi-institutional benchmarking and analysis of this generative artificial intelligence tool to investigate assessment integrity. European Journal of Engineering Education, 48(4), 559–614. https://doi.org/10.1080/03043797.2023.2213169
https://www.tandfonline.com/doi/full/10.1080/03043797.2023.2213169
Nikolic, S., Sandison, C., Haque, R., Daniel, S., Grundy, S., Belkina, M., … Neal, P. (2024). ChatGPT, Copilot, Gemini, SciSpace and Wolfram versus higher education assessments: an updated multi-institutional study of the academic integrity impacts of Generative Artificial Intelligence (GenAI) on assessment, teaching and learning in engineering. Australasian Journal of Engineering Education, 1–28. https://doi.org/10.1080/22054952.2024.2372154
https://www.tandfonline.com/doi/full/10.1080/22054952.2024.2372154