Democracy in Engineering Education The underlying question "Crocodile or Octopus?" was not properly answered during…
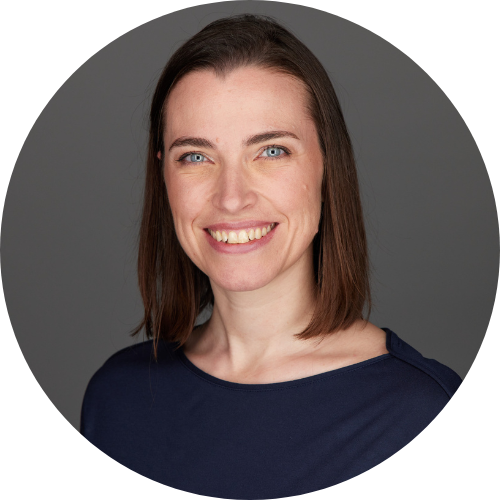
A paradigm shift has occurred in the discourse around the influence of Artificial Intelligence (AI) on our livelihood over the past year 2022 due to the release of multiple Generative AI-tools i.e., tools that generate audio/visual/text-based content using large datasets of the same media. OpenAI’s ChatGPT 3.5 release in particular has caused a stir in engineering universities across the world because of the portended implications these technologies may have on campus – should students be allowed to use ChatGPT for coding and design assignments? Should educators use AI avatars to deliver video lectures? Should administrators institute AI-based grading systems to produce feedback for student work?
It is important to acknowledge that there will be a definite impact of Generative AI on education, with several potential benefits for both teaching and learning. However, it is also imperative to separate exaggerations of their capabilities – catastrophic dangers (“doom”) or unimaginable utopias (“hype”) – from the current, pragmatic ethical risks these technologies already pose. The use of Generative AI in engineering education must encompass all of these themes on both macro and micro levels. On the one hand, on the macro level, students and teachers must analyse Generative AI for education based on their benefits (how and whom does it benefit?) as well as the ethical issues pertaining to their use in a global sense (who pays the price for the benefits that it promises?). On the other hand, the micro level focuses on them as users in the classroom, giving them the space to recognize that Generative AI is merely a piece of technology with certain advantages to ease the teaching and learning process. At the same time, the micro level helps teachers and students flag ethical risks to themselves.
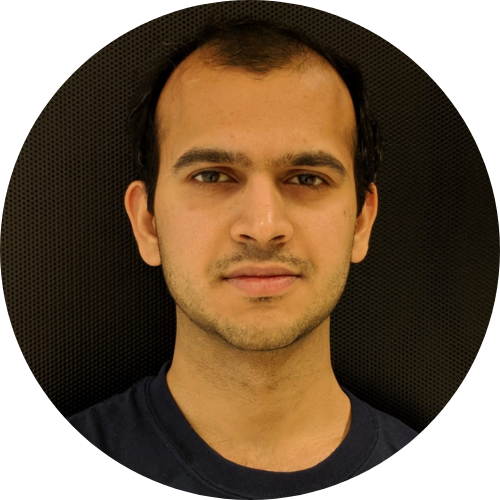
Unfortunately, at the micro and even more at the macro level, evaluating ethical risks has been shown to be particularly challenging for novices – and we are all more or less novices when it comes to Generative AI. In this editorial, we propose to use the Digital Ethics Canvas (Hardebolle et al., 2023), a framework designed for helping engineers identify ethical risks with respect to a digital solution under design, and apply it to the use of Generative AI in Engineering Education. This framework offers six ethical perspectives for performing a benefit-risk analysis, two of which we will explore in more detail: non-maleficence, which looks in particular at safety issues related to both intended and unintended use, and sustainability, which looks at environmental impact among other aspects. We selected these two perspectives because we feel that they should be discussed more widely when thinking about Generative AI in engineering educational settings.
Non-maleficence/safety: what kind of impacts can errors from the tool have?
A very common use case for ChatGPT, especially for students, is to pose questions to learn something new or seek new information. More generally, one expected benefit of AI-powered chatbots is to offer real-time tutoring to students at scale. However, text-based generative tools have an inherently severe limitation with respect to this type of task: they generate false information that appears very convincing. We will call this phenomenon “Plausible Nonsense” instead of the frequently used term “hallucination” as it can be misleading, both because the underlying phenomenon is different from human hallucinations and because the term conveys the idea that these systems “think” like humans. For use in an educational context, Plausible Nonsense is an issue to be taken seriously. But what is the risk exactly?
One reason that makes Plausible Nonsense very hard to detect is that humans tend to overly trust automated systems – a long documented phenomenon called automation bias (e.g. see Suresh et al., 2020). Unfortunately, all tools generating text with artificial neural networks share this limitation to different extents, including translation tools (e.g. Xu et al., 2023), summarization tools (e.g. Choubey et al., 2023), etc. Although the occurrence of Plausible Nonsense has reduced in more recent models, it is unclear when, or even if, the issue of Plausible Nonsense can be fixed.
In the short term, an important part of the problem is that generative tools generally do not provide ways for users to assess the correctness of the information that is presented – beyond warning messages upon login. Of course, the extent of the risks posed by Plausible Nonsense depends on the use case: it can be considered beneficial when generating ideas or poetry, but harmful when learning something new or when giving feedback to students. Educating users to the issue of automation bias, cultivating a critical view of AI-generated text, and being selective in the type of use that we recommend to students for generative tools are three approaches that have potential to reduce the risk.
Sustainability/environmental impact: what is the carbon footprint of these tools?
Some claim that generative tools can help address the complex issues related to climate change (“AI for green”) (Larosa et al., 2023), which is of immediate relevance to engineering education. At the same time, a growing body of literature warns about the massive amounts of resources that are expended to operate these tools (Bender et al., 2021). How does the environmental footprint of generative AI compare with other digital solutions that are used daily in (engineering) education?
In a widely cited study, Patterson et al. (2021) report that training GPT3, the precursor of ChatGPT, produced 552 metric tons of CO2 equivalent (Patterson et al., 2021). Using estimations based on Google data, this would be similar to around 143.4 million Google Search interactions (2 queries and 3 page views amounting to 3.85g CO2e / interaction). It would also be roughly equivalent to 32.5 million emails (long email at 17g CO2e / email), 20 million hours of video streaming on YouTube (27.6g CO2e / hour) or 3.5 million hours of Zoom call with video on (157g CO2e / hour) according to other sources.
Unfortunately, this is only part of the picture. Depending on estimations, training the AI may only account for approximately 20% of the tool’s total carbon footprint; the bulk of the impact comes from its usage (Patterson et al., 2021). The lack of data on the environmental cost of this usage is currently a major challenge in the field. And carbon footprint is not the only environmental cost to take into account: other studies also suggest that GPT-3’s total freshwater footprint for training could be around 3.5 million litres (Li et al., 2023).
Although we are still in the early stages of this type of research and data is still lacking, these estimates tend to indicate that generative tools are among the least “environmentally friendly” digital technologies. To add some nuance, it is worth highlighting that there are other types of AI models similar to GPT3 that have a much lower environmental impact according to the studies above, so the choice of the tool makes a difference. Another important criterion in selecting AI tools is understanding that the scale of environmental impact largely depends on the type of equipment and the geographical location of the servers running the software. In conclusion, carefully assessing the value added by generative tools compared to other less impactful teaching and learning tools and taking environmental concerns into account in the choice of providers is essential.
ConclusionThere are benefits to be expected from Generative AI in engineering education and in many sectors where these tools have the potential to augment, rather than automate and replace, human work (Eloundou et al., 2023). At the same time, these technologies have been – and are still being – released to the public hastily without providing sufficient safeguards for ethical implications that could have been anticipated. For instance, AI detectors, which are supposed to differentiate text written by ChatGPT from genuine student productions, have recently been shown to be biassed against non-native English writers (Liang et al., 2023), which is particularly concerning for European education. Our goal in introducing tools such as the Digital Ethics Canvas is to allow educators, students and administrators to have nuanced conversations that balance the benefits with the risks, without inducing moral panic. This editorial is not meant to discourage the use of AI tools, but to critically examine their use, especially in engineering classrooms. Just like with any other digital tool, we should renew the habit of examining the values carried by the tools we introduce in our classrooms and assess whether they align with the values we want to reflect in our educational practice. In that sense, the debate on the ethical issues with Generative AI is useful in making these value tensions visible again.