Democracy in Engineering Education The underlying question "Crocodile or Octopus?" was not properly answered during…
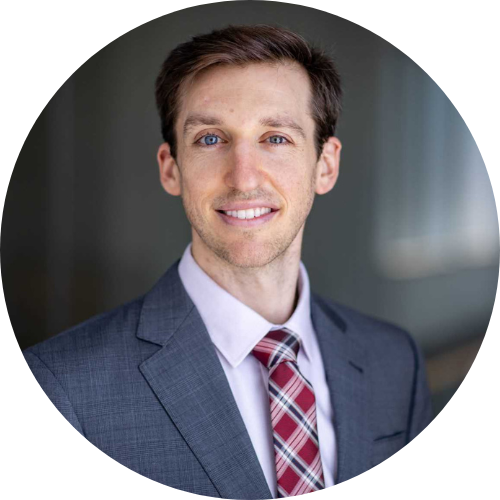
Almost one year ago, OpenAI released ChatGPT for public use. In the following months, other large organizations started releasing competitor large language models for text generation. To some observers, these technologies opened a Pandora’s box. Downsides from the perspective of educators include concerns about academic integrity, misplaced incentives, and general challenges to think about how best to react to generative text models. There is also understandable excitement. For example, Khan Academy is exploring what these models might mean for personalized learning through intelligent tutoring systems that can help students through better metaphors and explanations that resonate more with each learner. They call their system Khanmigo.
In my own work, I focus on trying to understand what these models can and cannot do to support engineering education teaching and research. I especially focus on areas within educational assessment where open-ended questions and prompts that instruct students (or research participants) to write their response to a question can lead to better information than multiple choice items but historically may be underutilized because they also require more time to analyze and grade. Some of the areas where we have investigated the models’ abilities include identifying themes in student essays, ethics case studies, student teammate feedback, or exam wrappers. In these studies, we follow a similar pattern of characterizing how transformer-based neural networks perform when categorizing what students are writing in short (50-100 words) and medium-length (100-500 words) essays. The ultimate goal is to know if these models can reliably generate and apply qualitative codebooks to variable-length data we often collect in engineering education teaching and research. This translates to an exercise in trying to minimize uncertainty in what the models are doing so that one might be more confident if these were used in live educational assessment settings.
In our work, we have found that in many cases the generative models perform as well as human analysts when identifying themes in writing as long as the prompts are not ambiguous. Admittedly, that is a non-trivial condition because prompts to generative text modeles – the instructions that one sends to the model to instruct it on how it generates its output – can easily be misleading and send the model down an unintended path. This underscores the importance of clear communication in assessment more generally in order to have clear signals from students of their knowledge, skills, and abilities. Along with susceptibility to suboptimal prompts from ambiguous communication, other limitations to these models can include the black-box nature of the models themselves, environmental impacts of training and running the models, and alignment – aligning the model’s objectives with those of the user and/or other stakeholders. This means that although the models can be useful, their use requires caution and judicious employment rather than unabashed adoption. At a minimum that most likely will look like lessons on prompt engineering best practices to improve the quality of model generation and digital information literacy to improve the ability to critique model output.
Cautionary Note Regarding Impacts on Assessment
As one can tell from that list, I spend a lot of time thinking about the impact of these technologies on classroom assessment. One study from Nikolic et al. earlier this year demonstrated how initial versions of generative models were already capable of completing various common types of assessments in engineering education. As these models become more refined in the future, faculty members will need to consider what they are trying to achieve through assessment. That raises the general question of how engineering faculty members may define assessment – assuming those definitions then drive the purposes one has for those assessments. In a survey of 142 engineering faculty members at universities across the United States, we found a wide variety in how they define assessment. So, maybe for some faculty members these models will have minimal impact; for most others, however, one should anticipate a non-trivial impact. This is especially true as the models transition from being text-to-text generative models and toward multimodality – allowing image input, for example. At that point, I suspect the conversation about the roles of generative AI in engineering education will become even more salient than they currently appear to be. Although these models might help us reduce uncertainty in students’ knowledge, skills, and abilities as we continue to develop assessment practices, they also introduce complications from students potentially using them to assist with the assessments – where generative AI models may help reduce uncertainty in some ways, they may introduce uncertainty in others.
Extra material
Impacts on Engineering Work
As we reconsider assessment, we may also need to reconsider what we are trying to assess. This begs the question of whether/how generative models would impact engineering practice. Those impacts could range from communication to design.
Notable Limitations
Finally, before concluding, I believe it is necessary to emphasize the standard caveats about these models and their limitations. Commonly cited ones include bias and hallucinations. They also have nontrivial environmental impacts. There are additional concerns about equitable access, economic impacts, and political bias.