Democracy in Engineering Education The underlying question "Crocodile or Octopus?" was not properly answered during…

ChatGPT and other generative Artificial Intelligence (GenAI) tools are having a massive impact on many different aspects of society, causing alarm and excitement in equal measure. Its powerful creative abilities are challenging established norms and undermining trust in social and cultural institutions. Lawyers have been caught using spurious references hallucinated by ChatGPT in a court filing; artists, writers, and actors are protesting the use of AI in the creative and performing arts; and with the upcoming politically charged presidential election in the United States, the electoral commission is scrambling to regulate the use of so-called ‘deep fakes’ in political advertising. With ChatGPT arguably passing the Turing Test and seeming to demonstrate near-human reasoning, these and other concerns have led to calls for a pause on AI research from many tech and business leaders. The European Union is trying to get ahead of the game through the EU AI Act, the first regulation on artificial intelligence to ensure that AI systems used in the EU are safe, transparent, traceable, non-discriminatory, and environmentally friendly.
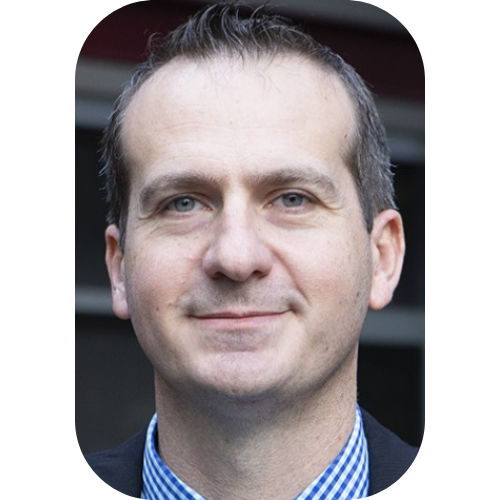
These concerns also extend to the education sector, and in particular, the validity and integrity of assessment. ChatGPT has recently passed the Bar Exam (used to qualify for legal practice), the United States Medical Licencing Examination, and a range of other assessments. With its generative power bound only to increase as it’s trained on larger and larger data sets, supported by faster back-end processing, governments (including those of Australia, Japan, and many other countries) are rushing to investigate and regulate AI in education.
In our recent work, we turned our attention to benchmarking the performance of ChatGPT in engineering education assessment by using it to complete all assessments for ten engineering subjects across seven universities. A SWOT analysis uncovered that much current practice is susceptible to integrity challenges, and while workarounds were identified, they are time-limited. But all is not lost, with many positive opportunities discovered and recommendations made. The challenge becomes how to adapt traditional teaching practices and transform assessment implementations to take advantage of the opportunities and minimise the risks. As engineers, we use technology to be more productive and sustainable, support our work to be more accurate and reliable, and reduce costs while ensuring safety. If used well, AI will be an enabler; therefore, turning our heads is not a viable option.
The question becomes, how do we put such vision into practice? There are now more questions than answers, so we need to put our heads together. The following are several starting points for such a discussion:
- How do we ensure Privacy, Data Security and Data Ownership to protect the student and institution?
- How do we explain, teach, and accommodate the Bias and Fairness problems associated with AI algorithms?
- In what ways do we use Transparency and Accountability factors in determining which AI tools we integrate into education?
- How do we ensure Equity and Accessibility so that no student is left behind?
- How do we ensure that Teachers and Students Control the educational process and ensure Accountability for Learning Outcomes?
- How do we protect Psychological Well-being when AI is used to over-monitor and assess student’s performance and behaviour?
- How should we assess written language fluency when online translation tools powered by AI can instantly generate well-written exposition with mother tongue fluency in any language of instruction?
- How do we design learning experiences that capitalise on AI’s strengths and help students understand its weaknesses, without inadvertently training them to be better prompt engineers?
- Finally, how do we enable Security Against Misuse of AI for unethical purposes?
These guiding questions are not exhaustive but provide a range of research starting points to shed light on the dark road ahead as we rediscover teaching and learning. As first steps we certainly encourage all readers to put their own assessments to the test by submitting the task instructions as prompts to ChatGPT (acknowledging the complex legal issues regarding intellectual property and genAI), to reach out to other educators in your networks so that we can navigate these challenges together, and to reflect critically on what “authentic” really means in the context of engineering education assessment.
Perhaps a more daunting step is exploring how to explicitly use genAI with students to support learning. Initial findings in a trial by the second author within a project-based subject have reinforced one of the key observations from our own study – that for students to use genAI effectively they need to already know what a quality output should look like. Those using AI tools blindly came up only with generic, inappropriate solutions, supported by hallucinated false references. While some effort was made in the trial to prepare students for using genAI, it became clear that there is a steep learning curve for both staff and students in developing new approaches to build on genAI’s potential pedagogical powers. Much of this learning will need to come from educators prepared to learn by doing and sharing their findings.
Our recent work highlights that we cannot stand still for long. Uncertainty leads to unintended consequences.